Speakers
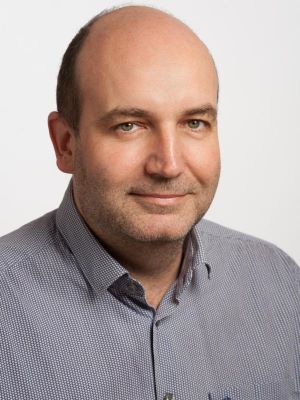
Dietmar Jannach, Professor
University of Klagenfurt, Austria
Bio:
Dietmar Jannach is a professor of computer science at the University of Klagenfurt, Austria. His main research theme is related to the application of intelligent system technology to practical problems and the development of methods for building knowledge-intensive software applications. In recent years, he worked on various topics in the area of recommender systems. In this area, he also published the first international textbook on the topic.
Title:
Recommender Systems, McNamara, and the Illusion of Progress
Abstract:
The field of recommender systems is flourishing. These systems are nowadays used on most major online sites where they can create significant value for both for consumers and providers. In parallel, hundreds of papers are published every year in computer science alone, and most of them report substantial advances to the state-of-the-art with the help of new machine learning algorithms. Recent studies however indicate that progress in this field might in fact be limited due to issues related to methodology and limited reproducibility. In this talk, we review some of these issues and outline possible directions for the future.
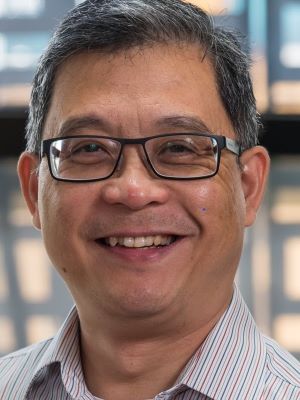
Guandong Xu, Professor
University of Technology Sydney, Australia
Bio:
Dr Guandong Xu is an Australian Computer Society (ACS) Fellow and the Professor at School of Computer Science, University of Technology Sydney, specialising in Data Science, Recommender Systems, and Social Computing. He has published 250+ papers in leading journals and conferences. He leads Smart Future Research Centre and Data Science and Machine Intelligence Lab at UTS. He is the Editor-in-Chief of Human-centric Intelligent Systems and assistant Editor-in-Chief of World Wide Web Journal and serving in editorial board or guest editors for several international journals. He has received several Awards from academia and industry, e.g., Top-10 Australian Analytics Leader Award and Australian Computer Society Disruptors Award.
Title:
Causal-based Recommender Systems
Abstract:
Causal learning has attracted a lot of research attention with the advance in explainable artificial intelligence. Causal learning contains causal discovery and causal inference two directions, where causal inference is to estimate the causal effects in treatment guided by causal graph structure and has been extended in tasks of counterfactual explanation, counterfactual fairness, disentanglement learning, interpretability, and debiasing. In this talk, we will introduce our latest research progress of incorporating causal learning into recommender systems, and present three recent studies on de-biasing confounding in recommendation, causal disentanglement for Intent Learning in Recommendation, and off-policy learning in recommendation. Experimental studies on real world datasets have proven the effectiveness of the proposed models.
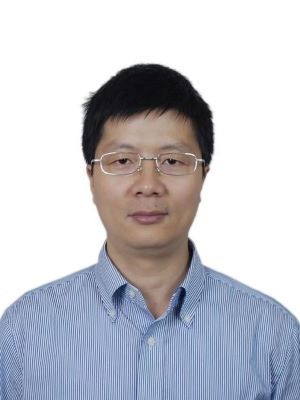
Jian Cao, Professor
Shanghai Jiao Tong University, China
Bio:
Computing is in the midst of a paradigm shift from individual computations to interaction. Current techniques are inadequate for network-based applications, which involve a number of heterogeneous (or independently designed) and autonomous (or independently operated) subsystems. The cooperation is an organized form of interation. My main areas of expertise are the developments of software and models to support coordination and cooperation among humans, systems and services. In recent years, my research interests include but not limit to:(1) Service Oriented Computing; (2) Network Computing; (3) Intelligent Data Analytics.
Title:
Towards Building a Fair Recommender Systems
Abstract:
With the wide applications of recommender systems, the potential impacts of recommender systems to the customers, item providers and other parties attract more and more attention. Fairness, which is the quality of treating people equally, is also becoming important in recommender system evaluation and algorithm design. Therefore, in the past years, there has been a growing interest in fairness measurement and assurance in recommender systems. In this talk, the concept of fairness will be discussed in detail in the various contexts of recommender systems. The framework to classify fairness metrics will be proposed from different dimensions. Then the strategies for eliminating unfairness in recommendations will be reviewed. Some research done by the team of the speaker will be presented as case studies. Finally, the challenges and future work will be discussed.
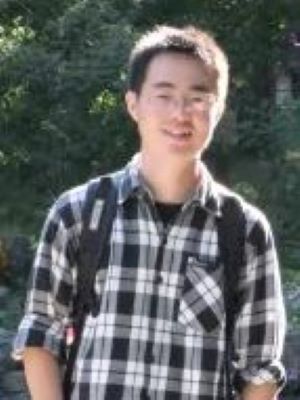
Fangzhao Wu, Senior Researcher
Microsoft Research Asia, China
Bio:
Fangzhao Wu is now a senior researcher at Social Computing (SC) group, Microsoft Research Asia. He joined MSRA in 2017. His research mainly focuses on natural language processing, user modeling and recommender systems. Fangzhao Wu received the Ph.D. and B.S. degrees both from Electronic Engineering Department of Tsinghua University in 2017 and 2012 respectively.
Title:
Personalized and Responsible News Recommendation
Abstract:
News Recommendation is critical for handling news information overload and improving users’ online news reading experience. It is widely used in many online news websites and Apps. Compared with traditional recommendation scenarios like e-commerce and movie recommendations, news recommendation has many special characteristics and challenges. In addition, the requirement of responsible news recommender systems becomes higher and higher. In this talk, we will introduce the goal, dataset, and benchmark of news recommendation, as well as the research on personalized and responsible news recommendation.